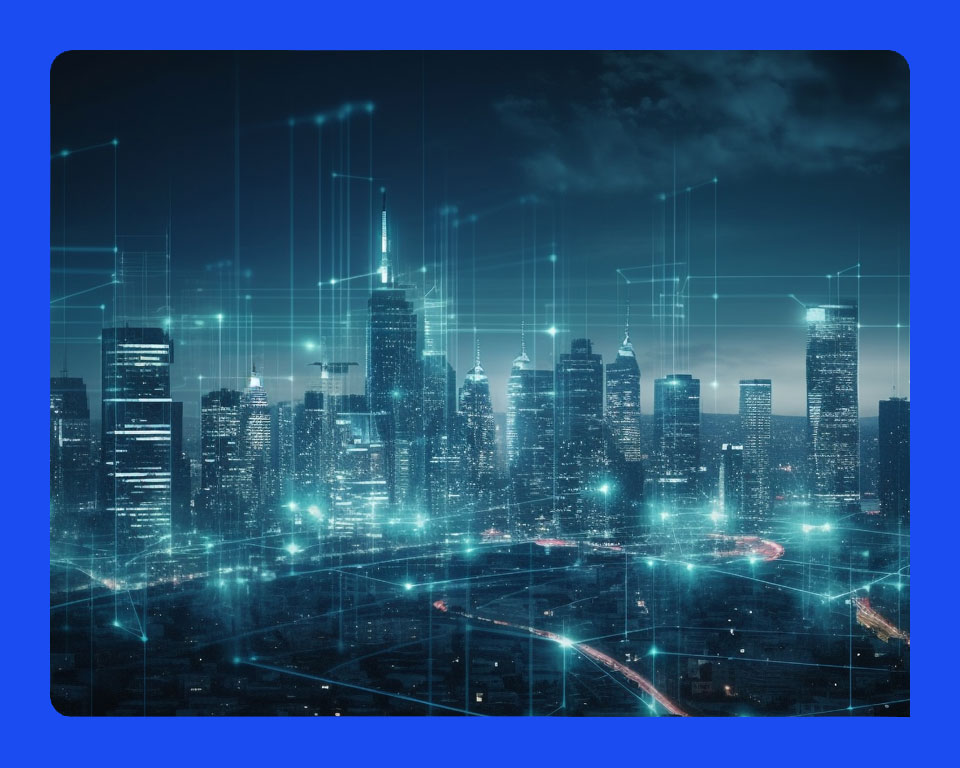
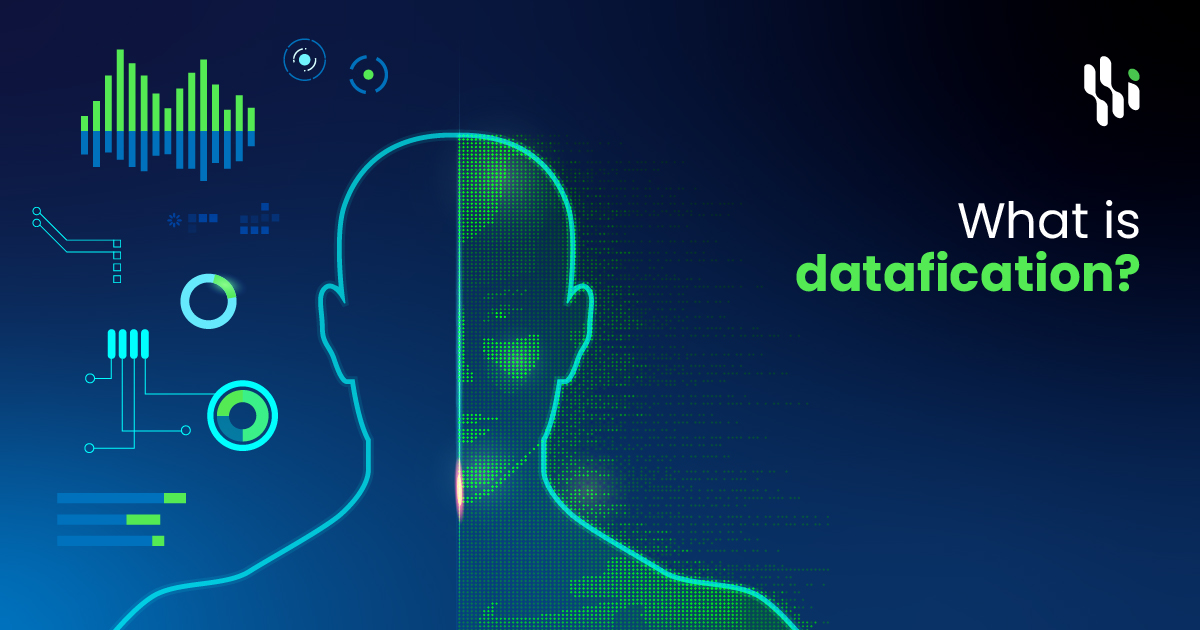
Over the past few years, “datafication” has become a popular term in the Big Data field. Although we hear the phrase “datafication” often these days, a quick online search is unlikely to turn up any relevant information about it. After researching the subject, though, most people are familiar with the concept, even if they have only sometimes used this particular phrase.
Datafication: What Is It?
The term “datafication” describes how the everyday activities of living things may be translated into a digital format and put to beneficial use in society. Datafication is measuring and recording social behavior to facilitate real-time monitoring and forecasting. In layperson’s terms, it is transforming an unseen process or action into data so that it can be observed, followed, analyzed, and optimized.
These days, there are many methods to “datify” even the most fundamental parts of our lives, thanks to the innovations made possible by modern technology. Datafication, in a nutshell, is a technical movement toward digitizing formerly analog operations to convert businesses into data-driven firms.
How Does Big Data Analysis Help in This?
It is no secret that Business Intelligence (BI) is becoming more popular in both the business and academic worlds. Although it is currently mostly seen through a technological lens, it is gradually expanding to include the data infrastructure, applications, tools, and best practices necessary for the efficient collection, representation, and distribution of data to influence decisions and actions.
Enterprise and social intelligence are merging as more and more decisions are made with an eye on shaping people’s actions in the future. Consequently, BI is a fertile basis for innovation, competitiveness, and productivity in the business sector. The academic community is starting to recognize the region’s growing significance and research potential.
Information gathering relies on data. Industry pundits generally characterize BI in the ‘3 Vs.’ as follows: Volume, Variety, and Veracity of Data.
The primary idea behind volume is that processing colossal data sets is advantageous; the underlying analytical premise is that more data is superior to better models. Scalability, dispersion, processing power, and similar factors are crucial here. According to the velocity hypothesis, the pace at which data flows is crucial, especially in light of the importance of the data flow rate in the feedback loop leading to action. It is essential to think about the granularity of your data streams, what you can safely throw away, and the acceptable latency in connection to your data, decisions, and actions.
Parting Thoughts
The theory of variety posits that real-world data is chaotic, including pieces often unrelated and presented in wildly varying formats that are prone to mistakes and inconsistency. The semantic integration and adaptability of the representation are other essential factors. Value, a newer concept, has arisen as a possible fourth, and its integration is crucial if we are to get any real benefit from our data.
One survey revealed that high-performing organizations are twice as likely to base their choices on in-depth analysis as low-performing ones, showing the broad perception that analytics provides value. However, leading companies increasingly rely on analytics, from long-term planning to operational tweaks.