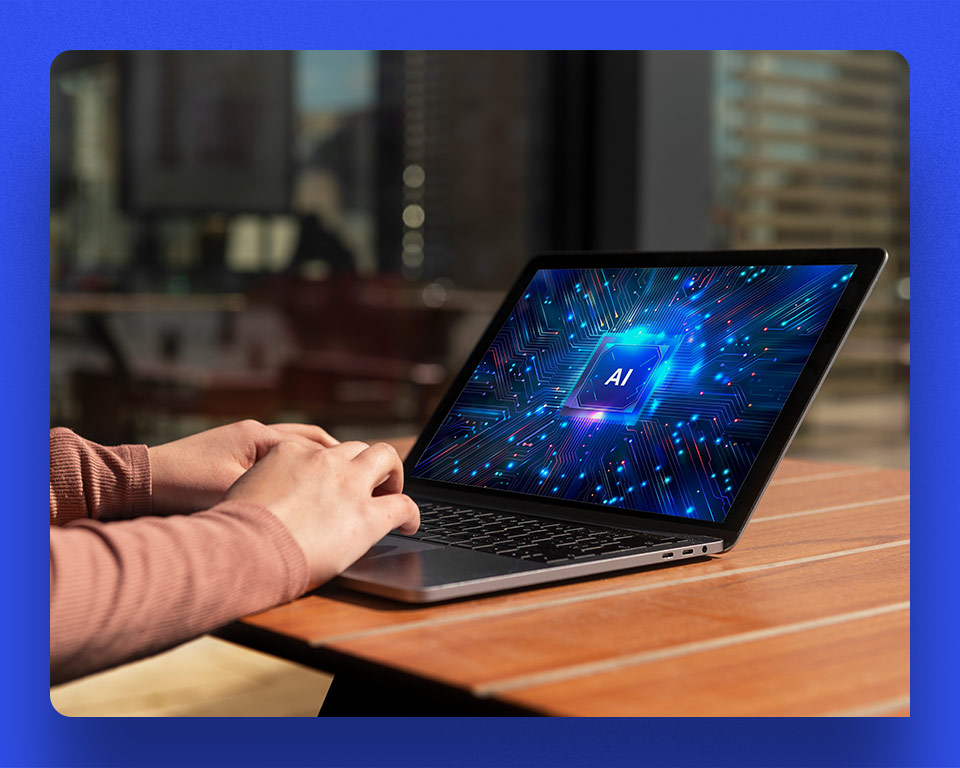
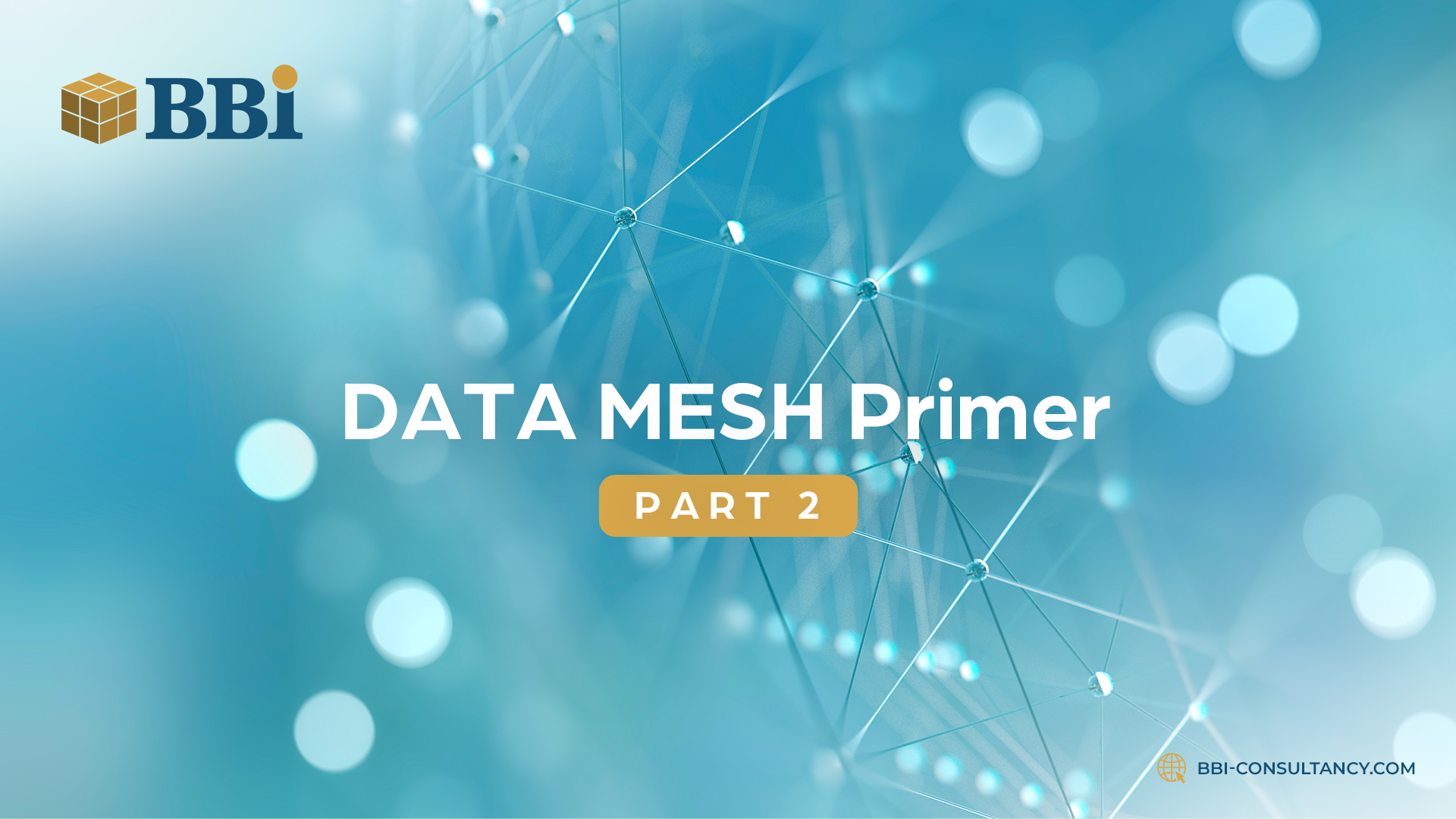
In part one of this series, we gave a general introduction to Data Mesh and why should organizations consider using this new technology. Today, we’ll continue exploring.
Data Mesh vs. Data Fabric
A data fabric is one approach to data integration that allows for data orchestration, while a data mesh follows a decentralized data architecture that distributes data into domains. While both are used in enterprise data management, they do have key differences, one of which is the human aspect.
Data fabric makes use of AI and machine learning to manage data, thus minimizing the need for human intervention. Meanwhile, a data mesh distributes data to domains, which are managed by their respective subject matter experts.
While both have overlaps, what you go with will depend on the type of approach you want to have. Data fabric has more of a top-down approach to data management, while the data mesh follows a bottom-up framework for enterprise data management.
Four Principles That Govern a Data Mesh
The data mesh operates on four principles:
- Decentralized data ownership and architecture that’s also domain-oriented
- Data as a product
- Self-service data infrastructure that doubles as a platform
- Democratic data governance (federated computational governance)
A successful data mesh leverages data governance, security, privacy, and federated data management.
Data Management Areas Improved by Data Mesh
- Data access
A data mesh’s decentralized data architecture eliminates data silos, therefore allowing better data autonomy, easier access to data, and interoperability.
- Data analytics
Its decentralized structure allows domains (teams) to access the data they need and work on it, reducing the time consumed by analytics processes.
- Data pipelines
The expansion of your data volume may require complex analytics projects, which can lead to equally complex data pipelines. A data mesh lets you build custom data pipelines.
- Data health
A data mesh improves data observability and makes it easier for you to check your data health.
Data Mesh Use Cases
- Data management/ data continuity
Data mesh can improve efficiency and reduce IT or network backlogs and operational costs. When used for data management or data continuity, it can help reduce time-to-market rates and complex processes, as well as enhance domain agility.
- DevOps
Data mesh eliminates geographic access limitations and reduces latency.
- Event sourcing
Data mesh can also be used for enhanced event sourcing, which can help you improve data governance.
- AI and machine learning
Data mesh can be used to create data warehouses that you can use to help AI and machine learning tools develop.
- Sales and marketing
Data mesh’s architecture can provide you with a holistic view of your customers, from their profiles to their behaviors. You can use this 360-degree view to enable hypersegmentation, build targeted marketing campaigns, and improve customer satisfaction.
Potential Issues With Using a Data Mesh
Data mesh is a promising solution, but it may not always be the best solution for your needs. If you’re handling a large volume of data, then it’s worth considering using a data mesh.
However, before fully adopting a data mesh for enterprise data management, you need to consider the following factors:
- Your data sources (quantity or volume)
- Your data team (the size of each team)
- Data governance
- Your data ecosystem and current data infrastructure
- Your data domains
- The technologies you’re using
Furthermore, depending on your organizational capabilities, it may take some time for you to fully integrate a data mesh into your data infrastructure.
The bottom line is, data mesh is a valuable tool for enterprises looking to transform themselves into data-driven organizations. It goes beyond seeing data as just an asset; it lets you utilize and experiment with data and its management to help you scale your operations more efficiently.
In the next articles, we’ll take a look into things to consider before implementing datamesh.