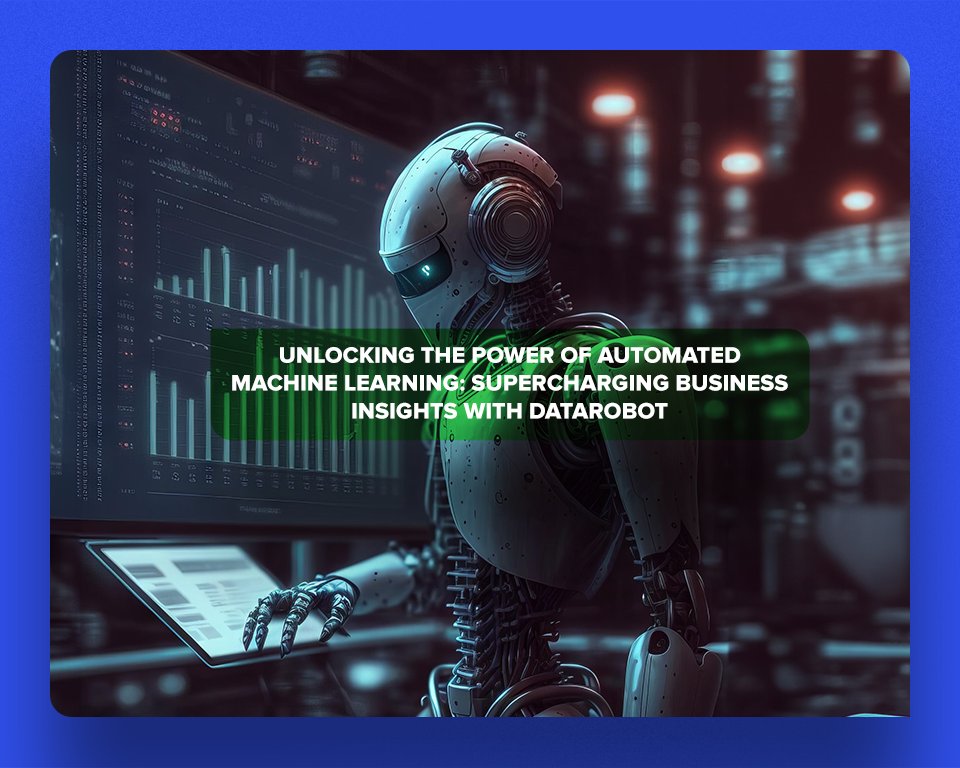
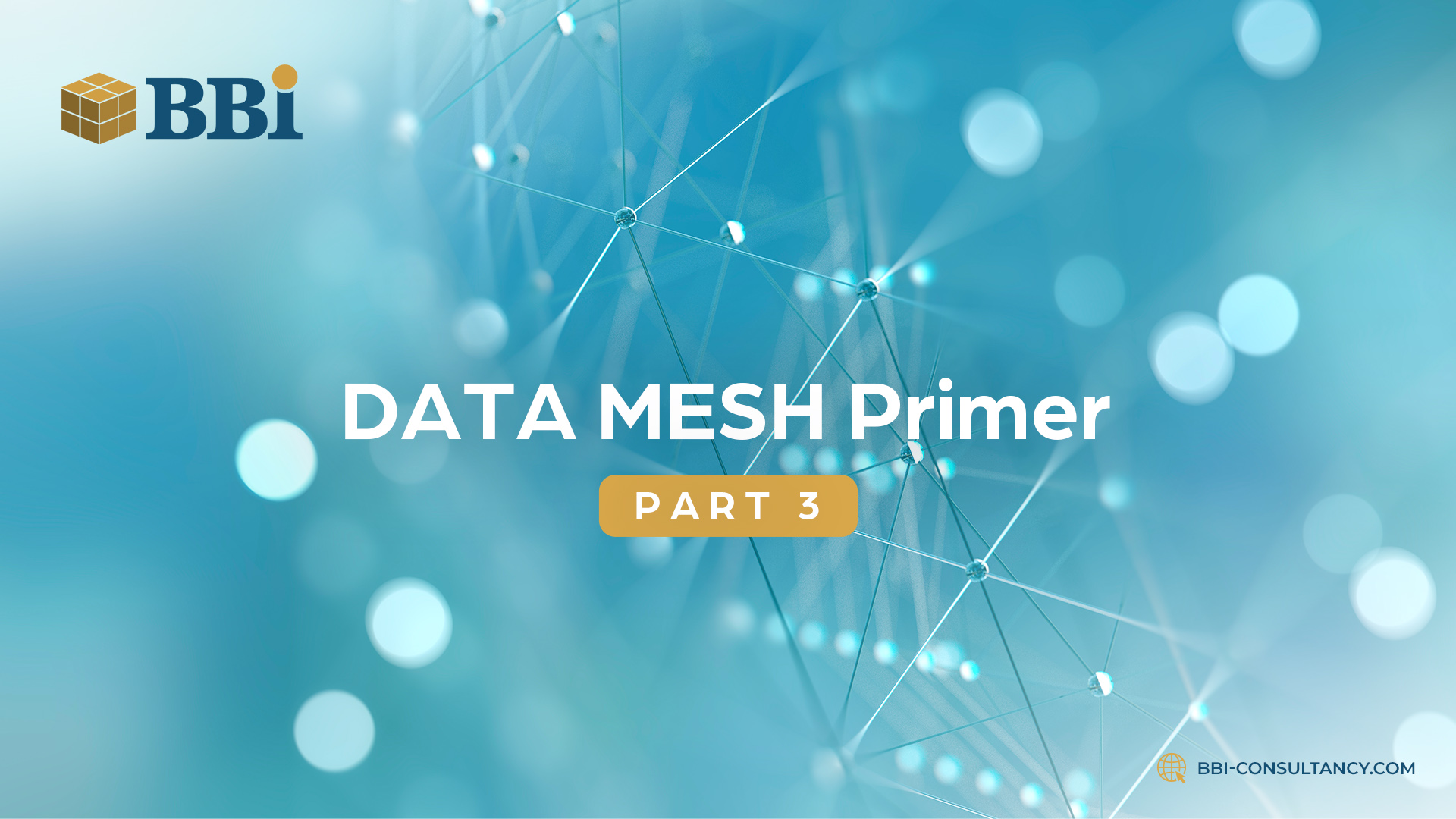
Before fully embracing data mesh for your organization, take note of a few key considerations. In this article, we’ll discuss the things you should take into account, as well as share real-life examples of companies that are leveraging the capabilities of data mesh.
Four Key Considerations Prior to Implementing Data Mesh Within Your Organization
1. Your business structure
You need to assess your organization’s structure before implementing data mesh. Ideally, your teams should be capable of working together instead of working in silos or vacuums. Additionally, you have to think about the size of your organization and the number of your domains so you can minimize potential issues that may arise about ownership.
Lastly, you need to factor in your business goals and initiatives. How will these align with your data mesh strategy? Your plans and objectives must be evaluated with your new strategy, so you can deliver measurable results and real value.
2. The need for a modern streaming engine platform
You will also need to have a modern engine streaming platform, such as Kafka, that can support the new productivity levels that come with using a data mesh architecture. However, it should still be able to let you have a self-service infrastructure. Using a streaming engine platform can help make your transition to data mesh smoother and addresses any gaps between different workloads.
3. Future-proofing technologies
The technology choices you’re making now will have an impact on how your new data mesh strategy will perform in the future. As such, you need to consider future-proofing your technologies, as well as your technology choices, to avoid building data products that will, in turn, cause you to rack up technical debt. You have to keep in mind that data mesh doesn’t involve one-time implementation. Thus, you will need to consider how it fits into your long-term digital transformation plan.
4. Challenges with decentralized architecture
- Data duplication. Duplicated data may happen when you’re integrating information across domains. As such, you need to account for this before adopting data mesh to minimize or prevent redundancy and spending.
- Data quality. If you’re handling your own integration, you need to consider your data product’s quality. Your domain team should oversee data and make quality checks to remove bad data.
- Schema co-location. Each domain in a data mesh should have separate data schemas to avoid any potential bottlenecks. However, you must also be able to integrate all of your data across different domains.
In the next part, we’ll investigate some major enterprises that implemented data mesh.